Water quality is crucial for human health, biodiversity, and ecosystem integrity. However, the ever-increasing list of pollutants, complex interactions between them, and their dynamic behaviour across spatial and temporal scales make water quality assessment a challenging task. Traditional water quality monitoring methods rely on manual collection of samples followed by laboratory analysis. While this approach is accurate, it is time-consuming and expensive, and cannot provide complete coverage of water bodies.
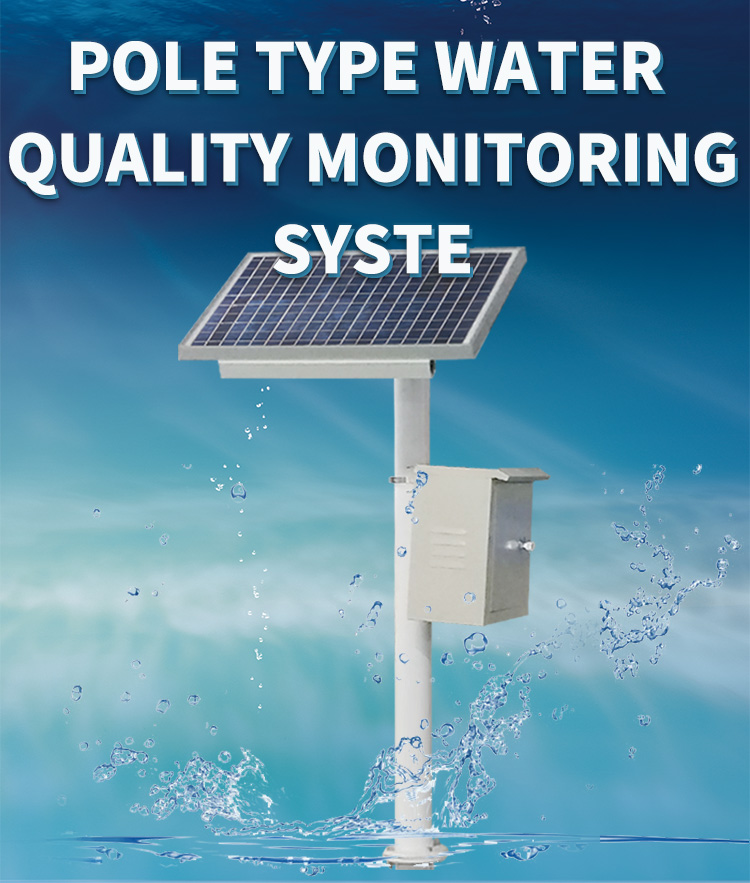
Recently, real-time water quality monitoring technologies, such as sensors, have gained popularity owing to their ability to measure multiple parameters simultaneously, assure continuous data collection, and transmit data quickly. Nevertheless, these devices have challenges with data management, and usually require expert knowledge for data interpretation. This makes the integration of machine learning algorithms and predictive models an appealing choice to enhance the accuracy and efficiency of water quality monitoring.
Machine learning (ML) is a branch of artificial intelligence (AI) that involves building statistical models from large amounts of data. ML algorithms can identify patterns in complex datasets, recognize relationships between various parameters, and forecast future trends based on historical data. These techniques are particularly suitable for water quality monitoring since they can handle high-dimensional datasets and account for spatio-temporal variability.
One vital application of ML in water quality monitoring is the prediction of pollutant concentrations before they exceed the regulatory limit. ML algorithms can learn from previous observations and environmental factors to forecast the likelihood of pollution events. For instance, a study conducted by Tang et al. (2021) used deep learning to predict the presence of fecal coliforms in rivers by combining hydroclimatic variables, land use maps, and drainage networks. Their results showed that the proposed method could produce more accurate predictions compared to conventional models, which rely solely on water quality data.
Another application of ML in water quality monitoring is anomaly detection. This involves identifying unusual measurements and raising alarms before the situation becomes problematic. Anomaly detection is particularly crucial in cases of accidental spills or hazardous events. ML algorithms can detect anomalies by comparing new measurements with historical data and detect variations that exceed a certain threshold.
ML techniques can also aid in data imputation, which involves filling missing or incomplete data with estimated values. This is essential for ensuring complete datasets before implementing predictive models. For instance, Wei et al. (2021) developed an ensemble model that integrates thermal infrared imaging and ML techniques to impute missing water temperature data in rivers. The proposed method demonstrated superior performance compared to traditional approaches, where missing data had to be manually filled in.
To conclude, incorporating ML algorithms and predictive models into water quality monitoring systems holds immense potential for enhancing accuracy, efficiency, and reducing costs while providing early warning systems of contamination risks. However, the implementation of ML models in real-world water quality monitoring systems should be carefully evaluated concerning factors such as the availability of data, computational resources, and interpretability of the results.