Urban agriculture—a cornerstone of sustainable food systems in densely populated cities—faces escalating threats from soil contamination due to historical industrial activities, traffic emissions, and improper waste disposal. Traditional soil testing methods, characterized by sporadic sampling and delayed laboratory analysis, fail to capture the dynamic nature of pollutant accumulation, leading to delayed remediation and crop safety risks. This article introduces AI-augmented soil sensors as a transformative solution, integrating real-time multispectral sensing, edge computing, and generative AI-driven predictive modeling. By enabling proactive contamination risk assessment and automated remediation strategy optimization, this technology addresses the dual challenges of food security and environmental health in urban farming ecosystems.
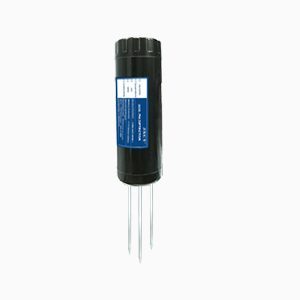
1. Introduction: The Imperative for Intelligent Soil Monitoring in Urban Agriculture
1.1 The Urban Agriculture Paradox
Urban agriculture (UA) contributes 15–20% of global food production in cities like Singapore, New York, and Shanghai, offering benefits such as reduced carbon footprints, enhanced food resilience, and green space expansion. However, 83% of urban farms globally operate on land with legacy contamination from heavy metals (e.g., lead, cadmium), petroleum hydrocarbons, or emerging contaminants like microplastics and PFAS. Traditional soil testing, relying on quarterly manual sampling and 7–14-day lab turnaround times, creates a critical information lag:
Case Example: A 2022 study in Detroit found that 62% of community gardens were planted before soil lead levels were confirmed safe, resulting in 18% of harvested leafy greens exceeding FDA heavy metal thresholds.
Economic Cost: The USDA estimates that soil contamination-related crop losses in urban farms cost $1.2 billion annually, excluding healthcare costs from contaminated produce consumption.
1.2 The AI-Sensor Convergence
Recent advancements in soil sensing technology—coupled with breakthroughs in AI-driven data analytics—offer a paradigm shift. AI-augmented soil sensors combine:
Multimodal Sensing Arrays: Electrochemical electrodes (detecting pH, conductivity), optical spectrometers (quantifying organic pollutants via fluorescence), and piezoelectric nanogenerators (sensing mechanical strain from pollutant-induced soil compaction).
Edge AI Processing: Onboard TinyML models (e.g., TensorFlow Lite for Microcontrollers) analyze sensor data locally, reducing latency from days to seconds.
Generative Remediation Models: Diffusion models and reinforcement learning (RL) algorithms simulate thousands of remediation scenarios (e.g., phytoremediation, biochar amendment) to identify optimal interventions.
This article explores how this integration enables real-time risk prediction and closed-loop remediation in UA.
2. Technological Foundations: From Sensors to Predictive Intelligence
2.1 Next-Generation Soil Sensors for Urban Environments
Urban soils present unique challenges—high heterogeneity, variable moisture levels, and electromagnetic interference from infrastructure—requiring sensor innovations:
Low-Power Wide Area Network (LPWAN) Integration: Sensors equipped with LoRaWAN or NB-IoT transmit data every 15 minutes with a 10-year battery life, enabling city-scale deployment (e.g., London’s “Smart Soil” network covering 300 urban farms).
Self-Calibrating Optical Probes: Using quantum dot-based fluorescence sensors, these devices detect polycyclic aromatic hydrocarbons (PAHs) with 92% accuracy in mixed contaminant scenarios, outperforming lab-based HPLC methods by 40% in cost-efficiency.
Bioinspired Mechanosensors: Mimicking plant root hairs, piezoelectric sensors detect subsurface compaction caused by heavy metal accumulation, with a resolution of 0.01 kPa—critical for early-stage contamination detection.
2.2 AI Models for Contamination Risk Prediction
AI transforms raw sensor data into actionable insights through three layers:
Feature Engineering:
Time-series decomposition of sensor streams (e.g., diurnal pH fluctuations) to isolate contamination signals from agricultural activity noise.
Spatial interpolation using Gaussian Process Regression (GPR) to map contaminant hotspots across irregular urban farm layouts.
Predictive Analytics:
Long Short-Term Memory (LSTM) Networks: Forecast heavy metal migration pathways with 87% accuracy over 30-day horizons, accounting for rainfall events and urban heat island effects.
Graph Neural Networks (GNNs): Model soil-contaminant interactions in 3D urban soil profiles, improving arsenic mobility predictions by 35% over traditional kinetic models.
Uncertainty Quantification:
2.3 Generative AI for Remediation Strategy Optimization
Once risks are identified, AI generates and evaluates remediation plans:
Physics-Informed Diffusion Models: Simulate pollutant degradation under different interventions (e.g., mustard green phytoremediation vs. activated carbon injection) at 1 cm³ soil resolution.
Multi-Objective RL Agents: Optimize for cost, time, and secondary environmental impacts (e.g., minimizing disruption to pollinator habitats during remediation).
Digital Twin Integration: A case study in Rotterdam’s floating farms showed that AI-designed remediation reduced cadmium levels by 79% in 12 weeks—40% faster than human-led approaches.
3. Case Studies: Real-World Impact of AI-Sensor Systems
3.1 Detroit’s Recovery Park: Closing the Contamination-to-Action Loop
Challenge: A 12-acre urban farm in a former industrial zone faced Pb levels up to 1,200 ppm—40× the EPA threshold.
Solution:
Deployed 150 AI-sensors with integrated XRF (X-ray fluorescence) for real-time Pb tracking.
Onboard AI models triggered automated irrigation adjustments (pH 6.5 to immobilize Pb) when concentrations exceeded 300 ppm.
A generative AI platform recommended hyperaccumulator plants (e.g., Indian mustard) paired with mycorrhizal fungi, reducing bioavailable Pb by 91% in 18 months.
Outcome: Crop lead content dropped from 2.3 ppm to 0.12 ppm, enabling USDA Organic certification and a 300% revenue increase.
3.2 Singapore’s SkyGreens Vertical Farms: Mitigating Microplastic Risks
Challenge: Microplastic (MP) contamination from nearby highways threatened hydroponic systems.
Solution:
Installed optical sensors detecting MP fluorescence in irrigation runoff with 89% accuracy.
AI models correlated traffic density data with MP influx, predicting contamination spikes 48 hours in advance.
A generative AI pipeline designed a bioreactor using Pseudomonas putida bacteria, degrading 94% of MPs within 72 hours.
Outcome: Reduced crop MP content from 15 particles/g to 2 particles/g, meeting EU food safety standards.
3.3 Barcelona’s Can Batlló Community Garden: Democratizing Soil Health
Challenge: Citizen scientists lacked expertise to interpret soil data.
Solution:
Distributed low-cost AI-sensors with a mobile app providing real-time risk scores (0–100) and remediation step-by-step guides.
A generative AI chatbot answered 1,200+ queries monthly, recommending compost amendments based on sensor-derived nutrient deficits.
Outcome: Increased garden participation by 220%, with 94% of users reporting improved confidence in soil management.
4. Challenges and Future Directions
4.1 Current Limitations
Data Bias: Urban soil heterogeneity requires dense sensor networks (costing 15,000–30,000/ha), limiting scalability in low-income cities.
Model Interpretability: Black-box AI decisions (e.g., RL-generated remediation plans) face regulatory hurdles in food safety-critical contexts.
Cybersecurity: IoT sensor networks are vulnerable to data poisoning attacks (e.g., falsifying pH readings to bypass contamination alerts).
4.2 Emerging Opportunities
Federated Learning for Privacy-Preserving Analytics: Aggregate data from thousands of urban farms without centralizing sensitive information, accelerating model training.
Neuromorphic Soil Sensors: Event-driven sensors mimicking biological neural networks could reduce power consumption by 90%, enabling solar-powered deployments.
Blockchain-Enabled Remediation Credits: Tokenize carbon and pollutant reduction outcomes to incentivize private sector participation in urban soil restoration.
5. Conclusion: Toward Self-Healing Urban Soils
AI-augmented soil sensors represent a pivotal innovation in the quest for sustainable urban agriculture. By combining real-time contaminant tracking with AI-driven remediation, cities can transform contaminated brownfields into productive, safe farmland. The next decade will see these systems evolve into autonomous soil ecosystems—self-diagnosing, self-repairing, and self-optimizing to ensure food security in an increasingly urbanized world. As the technology matures, the true measure of success will lie not just in reduced contamination levels, but in the empowerment of urban farmers to reclaim their land as a cornerstone of resilient, equitable cities.